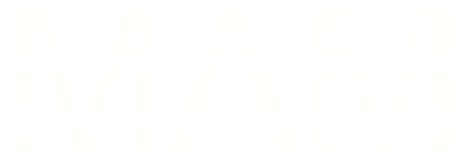
MAM is a prediction tool which is developed basing on deep learning algorithms. Majors provide those following several functions. To begin with, the MHC-CNN offer a high accuracy method to predict the affinity between specific MHC molecule and specific peptide. Moreover, we propose a MAM network for generating high-affinity peptides for a specific MHC molecule.
In this work, we propose the Motif Activation Mapping (MAM) network for MHC-I and peptides binding to extract motifs from peptides. Our MAM network is to calculate the contribution scores at each site then generate new peptides by mutating the amino acid with the lowest contribution score. Apart from it, we substitute amino acid randomly according to the motifs for generating peptides with high affinity.